생각하는 감쟈
[Python] 2-5) Matplotlib 2 본문
imshow
imshow 데이터 처럼 행과 열을 갖는 2차원 데이터는 imshow 명령으로 2차원 자료의 크기를 색깔로 표시
from sklearn.datasets import load_digits
digits = load_digits()#손글씨데이터
X = digits.images[0]
X
array([[ 0., 0., 5., 13., 9., 1., 0., 0.],
[ 0., 0., 13., 15., 10., 15., 5., 0.],
[ 0., 3., 15., 2., 0., 11., 8., 0.],
[ 0., 4., 12., 0., 0., 8., 8., 0.],
[ 0., 5., 8., 0., 0., 9., 8., 0.],
[ 0., 4., 11., 0., 1., 12., 7., 0.],
[ 0., 2., 14., 5., 10., 12., 0., 0.],
[ 0., 0., 6., 13., 10., 0., 0., 0.]])
plt.title("mnist digits; 0")
#interpolation='nearest' 보간법
# cmap=plt.cm.bone_r 색깔설정
plt.imshow(X, interpolation='bilinear', cmap=plt.cm.bone_r)
plt.xticks([])
plt.yticks([])
plt.grid(False)
#간격설정
plt.subplots_adjust(left=0.35, right=0.65, bottom=0.35, top=0.65)
plt.show()
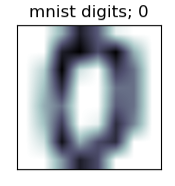
dir(plt.cm)[:10]
['Accent',
'Accent_r',
'Blues',
'Blues_r',
'BrBG',
'BrBG_r',
'BuGn',
'BuGn_r',
'BuPu',
'BuPu_r']
fig, axes = plt.subplots(1, 4, figsize=(12, 3),
subplot_kw={'xticks': [], 'yticks': []})
axes[0].set_title("plt.cm.Blues")
axes[0].imshow(X, interpolation='nearest', cmap=plt.cm.Blues)
axes[1].set_title("plt.cm.Blues_r")
axes[1].imshow(X, interpolation='nearest', cmap=plt.cm.Blues_r)
axes[2].set_title("plt.BrBG")
axes[2].imshow(X, interpolation='nearest', cmap='BrBG')
axes[3].set_title("plt.BrBG_r")
axes[3].imshow(X, interpolation='nearest', cmap='BrBG_r')
plt.show()
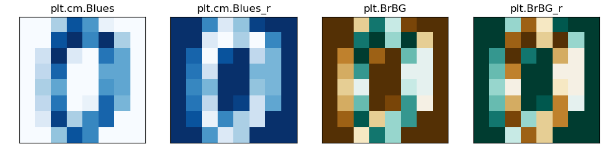
methods = [
None, 'none', 'nearest', 'bilinear', 'bicubic', 'spline16',
'spline36', 'hanning', 'hamming', 'hermite', 'kaiser', 'quadric',
'catrom', 'gaussian', 'bessel', 'mitchell', 'sinc', 'lanczos'
]
fig, axes = plt.subplots(3, 6, figsize=(12, 6),
subplot_kw={'xticks': [], 'yticks': []})
for ax, interp_method in zip(axes.flat, methods):
ax.imshow(X, cmap=plt.cm.bone_r, interpolation=interp_method)
ax.set_title(interp_method)
plt.show()
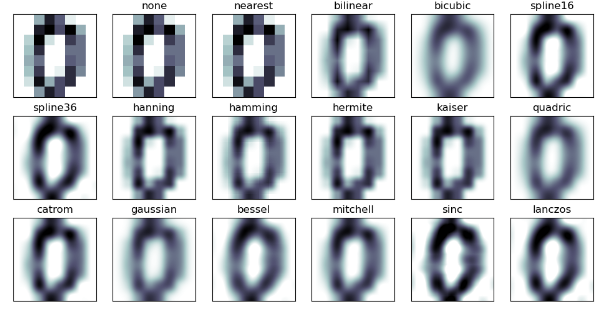
컨투어 플롯
입력변수가 x,y 두개가 출려변수가 z 하나인 3차원 자료
contour : 등고선 표시
contourf : 색깔로 표시
def f(x, y):
return (1 - x / 2 + x ** 5 + y ** 3) * np.exp(-x ** 2 - y ** 2)
n = 256
x = np.linspace(-3, 3, n)
y = np.linspace(-3, 3, n)
XX, YY = np.meshgrid(x, y)
ZZ = f(XX, YY)
plt.title("Contour plots")
plt.contourf(XX, YY, ZZ, alpha=.75, cmap='jet')
plt.contour(XX, YY, ZZ, colors='black')
plt.show()
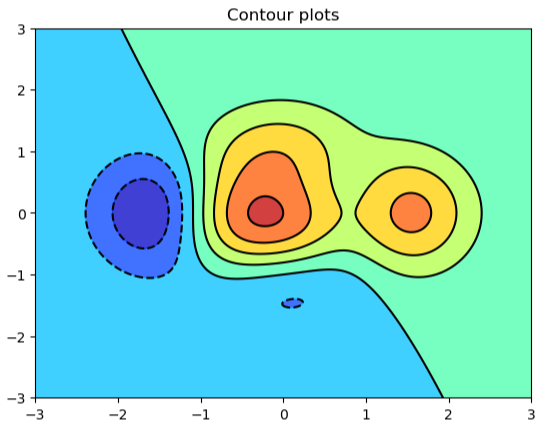
Seaborn을 사용한 데이터 분포 시각화
1.1 차원 분포 플롯
rugplot 데이터 위치를 x축 위에0 작은 선분으로 나타내어 실제 데이터들의 위치를 보여줌
kdeplot : 커널이라는 함수를 겹치는 방법으로 히스토그램보다 부드러운 형태의 분포 곡선을 보여줌
distplot : 러그와 커널 밀도 표시기능이 있음
import seaborn as sns
iris = sns.load_dataset("iris") # 붓꽃 데이터
titanic = sns.load_dataset("titanic") # 타이타닉호 데이터
tips = sns.load_dataset("tips") # 팁 데이터
flights = sns.load_dataset("flights") # 여객운송 데이터
import numpy as np
x = iris.petal_length.values
print(x)
sns.rugplot(x)
plt.show()
[1.4 1.4 1.3 1.5 1.4 1.7 1.4 1.5 1.4 1.5 1.5 1.6 1.4 1.1 1.2 1.5 1.3 1.4
1.7 1.5 1.7 1.5 1. 1.7 1.9 1.6 1.6 1.5 1.4 1.6 1.6 1.5 1.5 1.4 1.5 1.2
1.3 1.4 1.3 1.5 1.3 1.3 1.3 1.6 1.9 1.4 1.6 1.4 1.5 1.4 4.7 4.5 4.9 4.
4.6 4.5 4.7 3.3 4.6 3.9 3.5 4.2 4. 4.7 3.6 4.4 4.5 4.1 4.5 3.9 4.8 4.
4.9 4.7 4.3 4.4 4.8 5. 4.5 3.5 3.8 3.7 3.9 5.1 4.5 4.5 4.7 4.4 4.1 4.
4.4 4.6 4. 3.3 4.2 4.2 4.2 4.3 3. 4.1 6. 5.1 5.9 5.6 5.8 6.6 4.5 6.3
5.8 6.1 5.1 5.3 5.5 5. 5.1 5.3 5.5 6.7 6.9 5. 5.7 4.9 6.7 4.9 5.7 6.
4.8 4.9 5.6 5.8 6.1 6.4 5.6 5.1 5.6 6.1 5.6 5.5 4.8 5.4 5.6 5.1 5.1 5.9
5.7 5.2 5. 5.2 5.4 5.1]
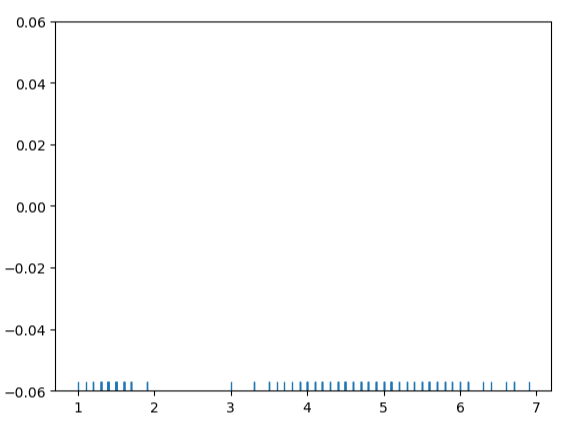
sns.kdeplot(x)#kernel density plot
plt.show()
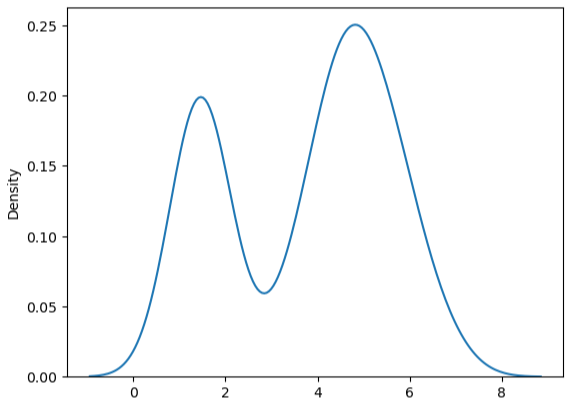
sns.distplot(x, kde=True, rug=True)
plt.show()
카운트 플롯
각카테고리 값별로 데이터가 얼마나 있는지 표시
sns.countplot(x="class", data=titanic)
plt.show()
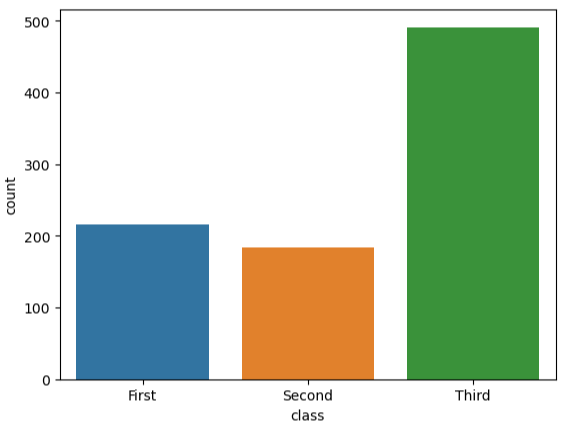
sns.countplot(x="day", data=tips)
plt.show()
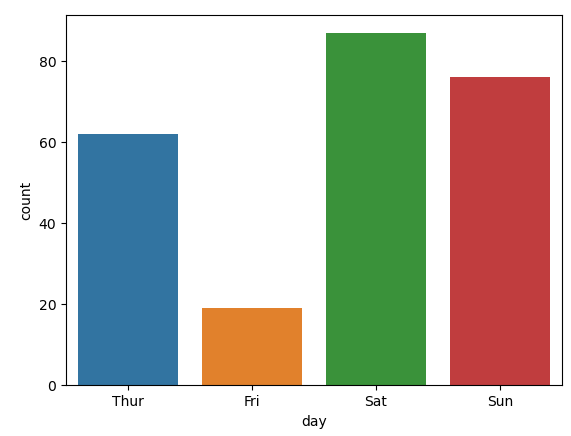
다차원 데이터
- 분석하고자하는 데이터가 모두 실수 값인 경우
- 분서하고자하는 데이터가 모두 카테고리 값인 경우
- 분석하고자하는 데이터가 모두 실수값과 카테고리 값이 섞여있는 경우
2차원 실수형 데이터
데이터가 2차원이고 모두 연속적인 실수 값이라면 스캐터 플롯 사용
iris.head()
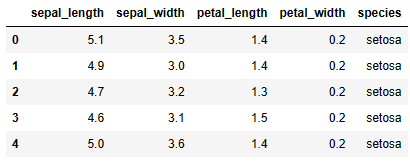
sns.jointplot(x="sepal_length", y="sepal_width", data=iris)
plt.suptitle("Joint Plot", y=1.02)
plt.show()
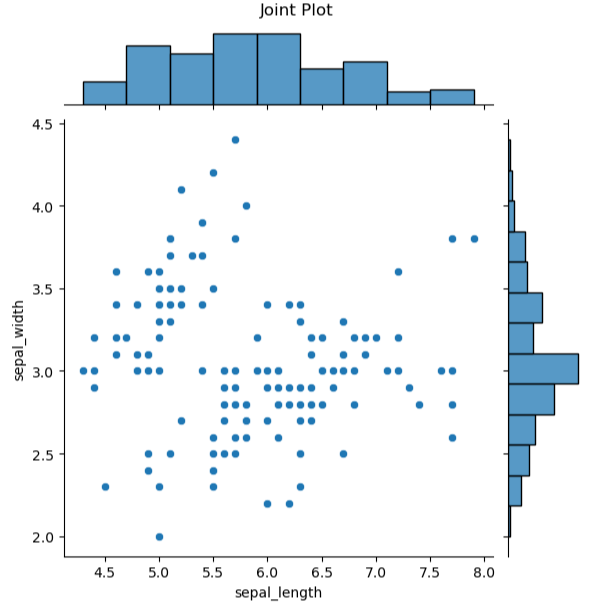
sns.jointplot(x="sepal_length", y="sepal_width", data=iris, kind="kde")
plt.suptitle("Joint Plot and Kernel Density Plot", y=1.02)
plt.show()
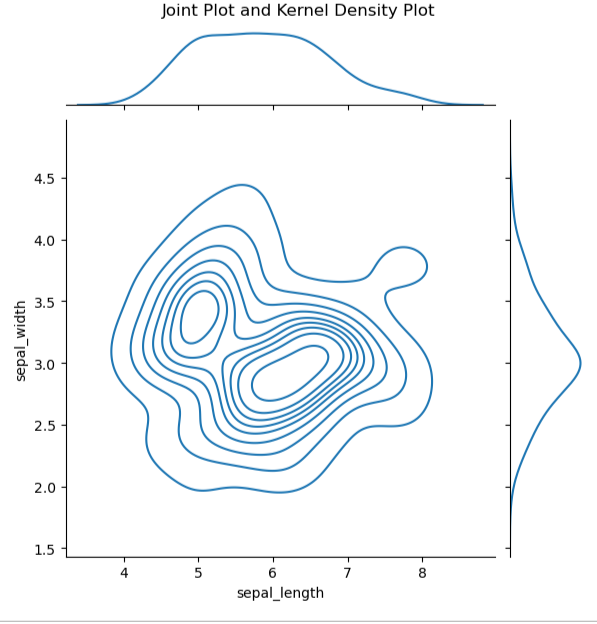
다차원 실수형 데이터
3차원 이상의 데이터라면 pairplot 명령
데이터 프레임을 인수로 받아 그리드 형태로 각 데이터 열의 조합에 대해 스캐터 플롯을 그림
대각선 영역에는 데이터 히스토드램을 그림
sns.pairplot(iris)
plt.title("Iris Data의 Pair Plot")
plt.show()
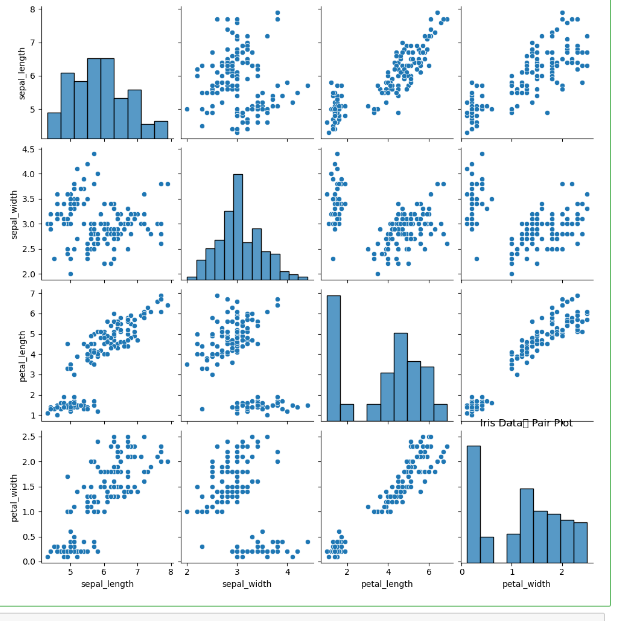
2차원 카테고리 데이터
데이터가 2차원이고 모든 값이 카테고리값일때 hearmap 사용
heat + map : 데이터들의 배열을 색상으로 표현
titanic_size = titanic.pivot_table(
index="class", columns="sex", aggfunc="size")
titanic_size
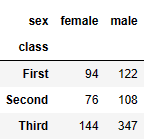
sns.heatmap(titanic_size, cmap=sns.light_palette(
"gray", as_cmap=True), annot=True, fmt="d")
plt.title("Heatmap")
plt.show()
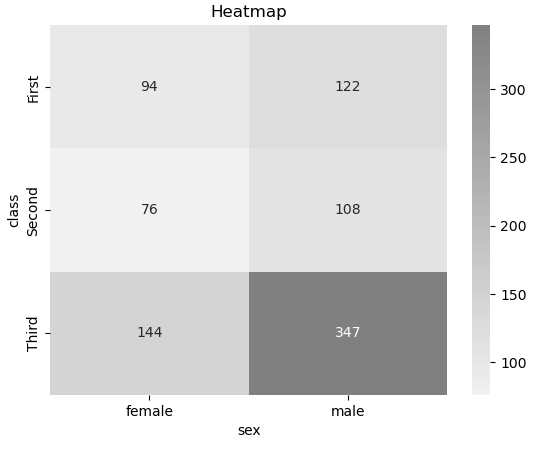
판다스의 시각화 기능
import pandas as pd
np.random.seed(0)
df1 = pd.DataFrame(np.random.randn(100, 3),
index=pd.date_range('1/1/2018', periods=100),
columns=['A', 'B', 'C']).cumsum()
df1.head()
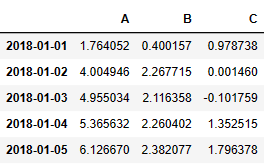
df1.plot()
plt.title("Plot of pandas")
plt.xlabel("time")
plt.ylabel("Data")
plt.show()
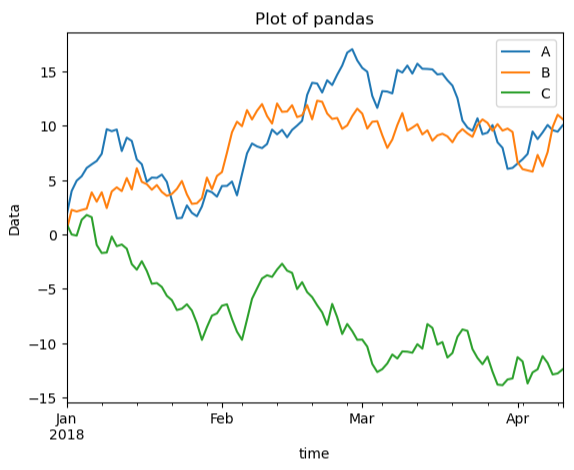
iris = sns.load_dataset("iris") # 붓꽃 데이터
titanic = sns.load_dataset("titanic") # 타이타닉호 데이터
#꽃받침 길이 시각화
iris.sepal_length[:20].plot(kind='bar', rot=0)
plt.xlabel("Data")
plt.ylabel("length")
plt.show()
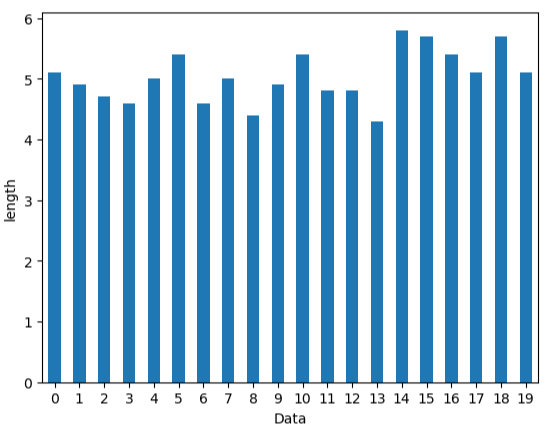
iris[:5].plot.bar(rot=0)#x축 라벨의 각도
plt.xlabel("Data")
plt.ylabel("Feature")
plt.ylim(0, 7)
plt.show()
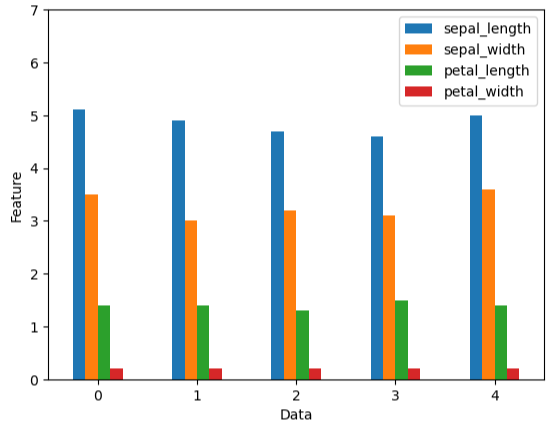
df2 = iris.groupby(iris.species).mean()
df2.columns.name = "feature"
df2
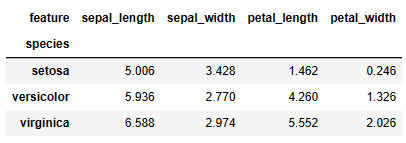
df2.plot.bar(rot=0)
plt.xlabel("mean")
plt.ylabel("kind")
plt.ylim(0, 8)
plt.show()
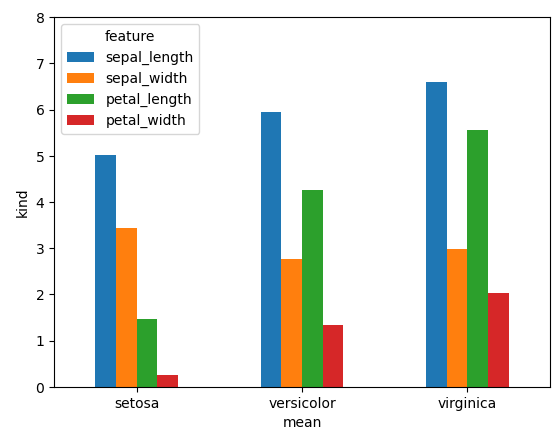
df2.T.plot.bar(rot=0)
plt.xlabel("Feature")
plt.ylabel("mean")
plt.show()
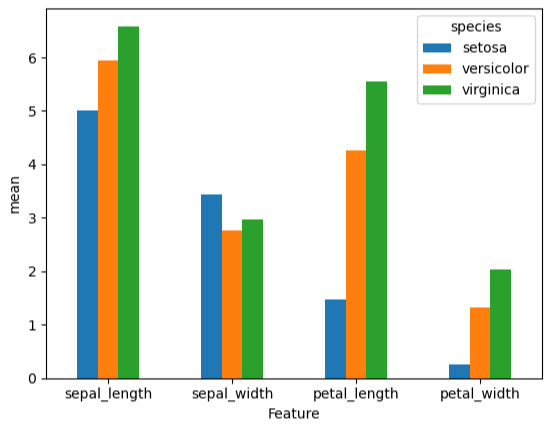
titanic[["pclass"]].head()
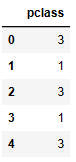
df3 = titanic.pclass.value_counts()
df3.plot.pie(autopct='%.2f%%')
plt.axis('equal')
plt.show()
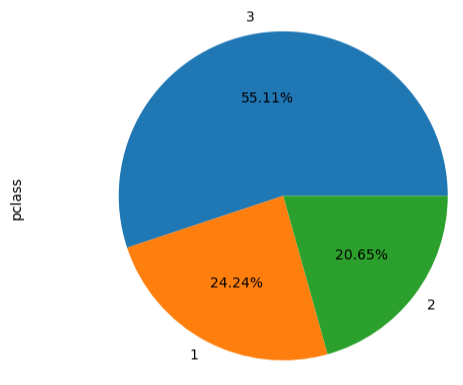
iris.plot.hist()
plt.xlabel("value")
plt.show()
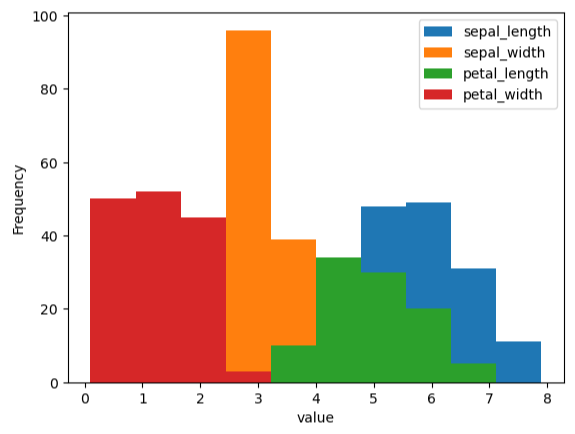
iris.plot.kde()
plt.xlabel("value")
plt.show()
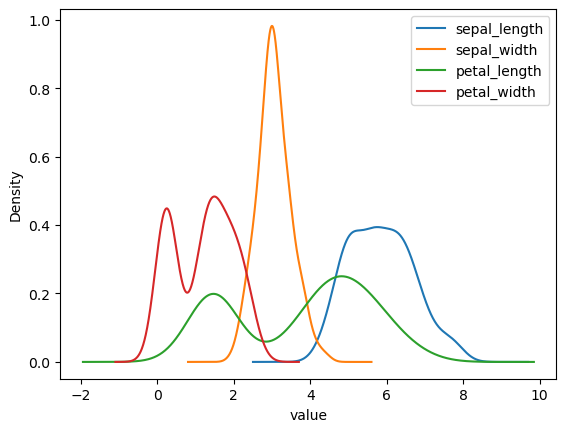
뭐지 numpy는 노잼이었는데
그래프는 왜 재미있냐...
난 공대가 맞았을ㅈ도....
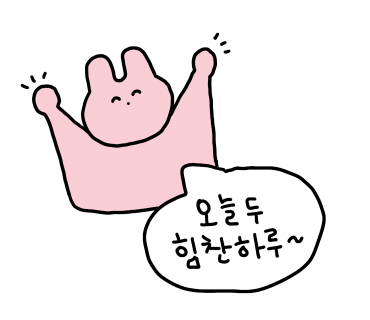
#ABC부트캠프 #부트캠프 #유클리드소프트 #파이썬 #고용노동부 #한국산업인력공단 #서포터즈 #python #Ai #Bigdata #cloud #대외활동 #대학생 #daily
'Language > Python' 카테고리의 다른 글
[Python] 3-2) 정규표현식, 워드클라우드 (0) | 2023.04.06 |
---|---|
[Python] 3-1) 데이터 수집 및 크롤링 (0) | 2023.03.30 |
[Python] 2-5) Matplotlib 1 (0) | 2023.03.29 |
[Python] 2-4) Data Frame 2 (1) | 2023.03.28 |
[Python] 2-4) Data Frame 1 (0) | 2023.03.28 |